InterSystems IRIS Scalability Overview
Today’s data platforms are called on to handle a wide variety of workloads. As a workload of any type grows, a data platform must be able to scale to meet its increasing demands, while at the same time maintaining the performance standards the enterprise relies on and avoiding business disruptions.
This page reviews the scaling features of InterSystems IRIS® data platform, and provides guidelines for a first-order evaluation of scaling approaches for your enterprise data platform.
Scaling Matters
What you do matters, and whether you care for ten million patients, process billions of financial orders a day, track a galaxy of stars, or monitor a thousand factory engines, your data platform must not only support your current operations but enable you to scale to meet increasing demands. Each business-specific workload presents a different challenge to the data platform on which it operates — and as a business grows, that challenge becomes even more acute.
For example, consider the two situations in the following illustration:
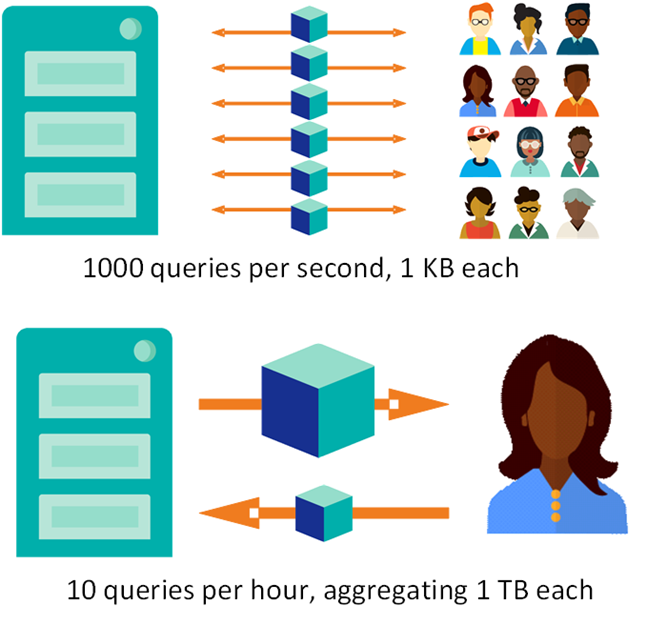
Both workloads are demanding, but it is hard to say which is more demanding — or how to scale to meet those demands.
We can better understand data platform workloads and what is required to scale them by decomposing them into components that can be independently scaled. One simplified way to break down these workloads is to separate the components of user volume and data volume. A workload can involve many users interacting frequently with a relatively small database, as in the first example, or fewer requests from what could be a single user or process but against massive datasets, like the second. By considering user volume and data as separate challenges, we can evaluate different scaling options. (This division is a simplification, but one that is useful and easy to work with. There are many examples of complex workloads to which it is not easily applied, such as one involving many small data writers and a few big data consumers.)
Vertical Scaling
The first and most straightforward way of addressing more demanding workloads is by scaling up — that is, taking advantage of vertical scalability. In essence, this means making an individual server more powerful so it can keep up with the workload.
In detail, vertical scaling requires expansion of the capacity of an individual server by adding hardware components that alleviate the workload bottlenecks you are experiencing. For example, if your cache can’t handle the working set required by your current user and data volume, you can add more memory to the machine.
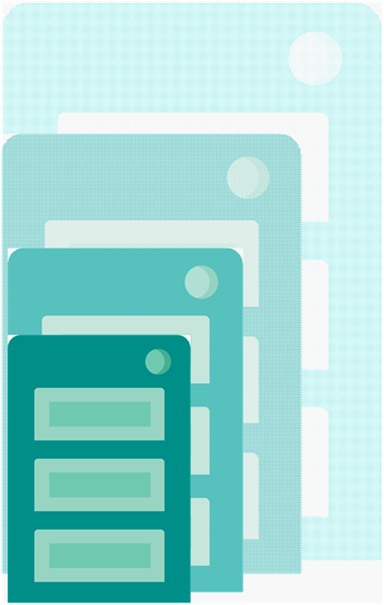
Vertical scaling is generally well understood and architecturally straightforward; with good engineering support it can help you achieve a finely tuned system that meets the workload’s requirements. It does have its limits, however:
-
Today’s servers with their hundred-plus CPU cores and memory in terabytes are very powerful, but no matter what its capacity, a system can simultaneously create and maintain only so many sockets for incoming connections.
-
Premium hardware comes at a premium price, and once you’ve run out of sockets, replacing the whole system with a bigger, more expensive one may be your only option.
-
Effective vertical scaling requires careful sizing before the fact. This may be straightforward in a relatively static business, but under dynamic circumstances with a rapidly growing workload it can be difficult to predict the future.
-
Vertical scaling does not provide elasticity; having scaled up, you cannot scale down when changes in your workload would allow it, which means you are paying for excess capacity.
-
Vertical scaling stresses your software, which must be able to cope effectively and efficiently with the additional hardware power. For example, scaling to 128 cores is of little use if your application can handle only 32 processes.
For more information on vertically scaling InterSystems IRIS data platform, see System Resource Planning and Management and General Performance Enhancement on InterSystems IRIS Platforms.
Horizontal Scaling
When vertical scaling does not provide the complete solution — for example, when you hit the inevitable hardware (or budget) ceiling — or as an alternative to vertical scaling, some data platform technologies can also be scaled horizontally by clustering a number of smaller servers. That way, instead of adding specific components to a single expensive server, you can add more modest servers to the cluster to support your workload as volume increases. Typically, this implies dividing the single-server workload into smaller pieces, so that each cluster node can handle a single piece.
Horizontal scaling is financially advantageous both because you can scale using a range of hardware, from dozens of inexpensive commodity systems to a few high-end servers to anywhere in between, and because you can do so gradually, expanding your cluster over time rather than the abrupt decommissioning and replacement required by vertical scaling. Horizontal scaling also fits very well with virtual and cloud infrastructure, in which additional nodes can be quickly and easily provisioned as the workload grows, and decommissioned if the load decreases.
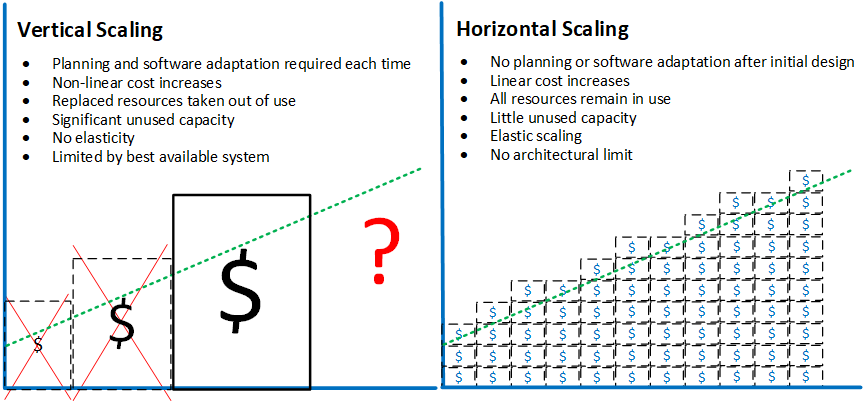
On the other hand, horizontal clusters require greater attention to the networking component to ensure that it provides sufficient bandwidth for the multiple systems involved. Horizontal scaling also requires significantly more advanced software, such as InterSystems IRIS, to fully support the effective distribution of your workload across the entire cluster. InterSystems IRIS accomplishes this by providing the ability to scale for both increasing user volume and increasing data volume.
Horizontal Scaling for User Volume
How can you scale horizontally when user volume is getting too big to handle with a single system at an acceptable cost? The short answer is to divide the user workload by connecting different users to different cluster nodes that handle their requests.
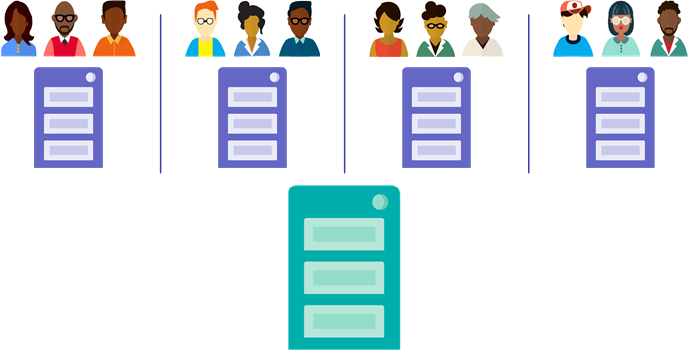
You can do this by using a load balancer to distribute users round-robin, but grouping users with similar requests (such as users of a particular application when multiple applications are in use) on the same node is more effective due to distributed caching, in which users can take advantage of each other’s caches.
InterSystems IRIS provides an effective way to accomplish this through distributed caching, an architectural solution supported by the Enterprise Cache Protocol (ECP) that partitions users across a tier of application servers sitting in front of your data server. Each application server handles user queries and transactions using its own cache, while all data is stored on the data server, which automatically keeps the application server caches in sync. Because each application server maintains its own independent working set in its own cache, adding more servers allows you to handle more users.
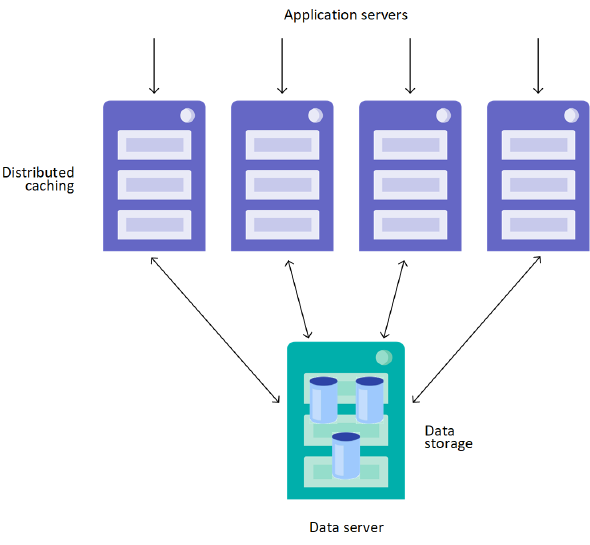
Distributed caching is entirely transparent to the user and the application code.
For more information on horizontally scaling InterSystems IRIS data platform for user volume, see Horizontally Scaling for User Volume with Distributed Caching.
Horizontal Scaling for Data Volume
The data volumes required to meet today’s enterprise needs can be very large. More importantly, if they are queried repeatedly, the working set can get too big to fit into the server’s cache; this means that only part of it can be kept in the cache and disk reads become much more frequent, seriously impacting query performance.
As with user volume, you can horizontally scale for data volume by dividing the workload among several servers. This is done by partitioning the data.
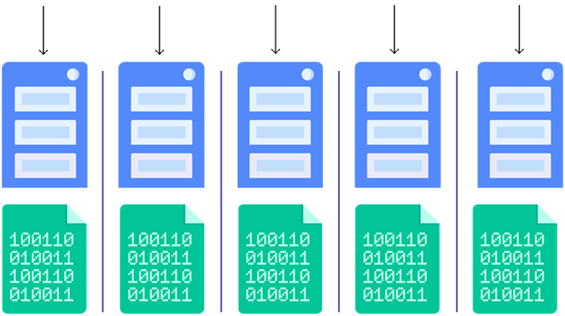
InterSystems IRIS achieves this through its sharding capability. An InterSystems IRIS sharded cluster partitions data storage, along with the corresponding caches, across a number of servers, providing horizontal scaling for queries and data ingestion while maximizing infrastructure value through highly efficient resource utilization.
In a basic sharded cluster, a sharded table is partitioned horizontally into roughly equal sets of rows called shards, which are distributed across a number of data nodes. For example, if a table with 100 million rows is partitioned across four data nodes, each stores a shard containing about 25 million rows. Nonsharded tables reside wholly on the first data node configured.
Queries against a sharded table are decomposed into multiple shard-local queries to be run in parallel on the data nodes; the results are then combined and returned to the user. This distributed data layout can further be exploited for parallel data loading and with third party frameworks.
In addition to parallel processing, sharding improves query performance by partitioning the cache. Each data node uses its own cache for shard-local queries against the data it stores, making the cluster’s cache for sharded data roughly as large as the sum of the caches of all the data nodes. Adding a data node means adding dedicated cache for more data.
As with application server architecture, sharding is entirely transparent to the user and the application.
Sharding comes with some additional options that greatly widen the range of solutions available, including the following:
-
Mirroring
InterSystems IRIS mirroring can be used to provide high availability for data nodes.
-
Compute nodes
For advanced use cases in which low latencies are required, potentially at odds with a constant influx of data, compute nodes can be added to provide a transparent caching layer for servicing queries. Compute nodes support query execution only, caching the sharded data of the data nodes to which they are assigned (as well as nonsharded data when necessary). When a cluster includes compute nodes, read-only queries are automatically executed on them, while all write operations (insert, update, delete, and DDL operations) are executed on the data nodes. This separation of query workload and data ingestion improves the performance of both, and assigning multiple compute nodes per data node can further improve the query throughput and performance of the cluster.
For more information on horizontally scaling InterSystems IRIS data platform for data volume, see Horizontally Scaling for Data Volume with Sharding.
Automated Deployment of Horizontally Scaled Configurations
InterSystems IRIS provides several methods for automated cluster deployment, which can significantly simplify the deployment process, especially for complex horizontal cluster configurations. For more information about automated cluster deployment, see Automated Deployment Methods for Clusters.
Evaluating Your Workload for Scaling Solutions
The subsequent topics cover the individual scalability features of InterSystems IRIS in detail, and you should consult these before beginning the process of scaling your data platform. However, the tables below summarize the overview, and provide some general guidelines concerning the scaling approach that might be of the most benefit in your current circumstances.
Scaling Approach | Pros | Cons |
---|---|---|
Vertical |
Architectural simplicity Hardware is finely tuned to workload |
Price/performance ratio is nonlinear Persistent hardware limitations Careful initial sizing required One-way scaling only |
Horizontal |
Price/performance ratio is more linear Can leverage commodity, virtual and cloud-based systems Elastic scaling |
Emphasis on networking |
Conditions | Possible Solutions |
---|---|
High multiuser query volume: insufficient computing power, throughput inadequate for query volume. |
Add CPU cores. Take advantage of parallel query execution to leverage high core counts for queries spanning a large dataset. |
High data volume: insufficient memory, database cache inadequate for working set. |
Add memory and increase cache size to leverage larger memory. Take advantage of parallel query execution to leverage high core counts. |
Other insufficient capacity: bottlenecks in other areas such as network bandwidth. |
Increase other resources that may be causing bottlenecks. |
Conditions | Possible Solutions |
---|---|
High multiuser query volume: frequent queries from large number of users. |
Deploy application server configuration (distributed caching). |
High data volume: some combination of:
|
Deploy sharded cluster (partitioned data and partitioned caching), possibly adding compute nodes to separate queries from data ingestion and increase query throughput (see Deploy Compute Nodes) |
General Performance Enhancement on InterSystems IRIS Platforms
The following information may be helpful in improving the performance of your InterSystems IRIS deployment.
-
Simultaneous multithreading
In most situations, the use of Intel Hyper-Threading or AMD Simultaneous Multithreading (SMT) is recommended for improved performance, either within a physical server or at the hypervisor layer in virtualized environments. There may be situations in a virtualized environment in which disabling Hyper-Threading or SMT is warranted; however, those are exceptional cases specific to a given application.
In the case of IBM AIX®, IBM Power processors offer multiple levels of SMT at 2, 4, and 8 threads per core. With the latest IBM Power9 and Power10 processors, SMT-8 is the level most commonly used with InterSystems IRIS. There may be cases, however, especially with previous generation Power7 and Power8 processors, in which SMT-2 or SMT-4 is more appropriate for a given application. Benchmarking the application is the best approach to determining the ideal SMT level for a specific deployment.
-
Semaphore allocation
By default, InterSystems IRIS allocates the minimum number of semaphore sets by maximizing the number of semaphores per set (see Semaphores in InterSystems Products). However, this is some evidence that this is not ideal for performance on Linux systems with non-uniform memory access (NUMA) architecture.
To address this, the semsperset parameter in the configuration parameter file (CPF) can be used to specify a lower number of semaphores per set. By default, semsperset is set to 0, which specifies the default behavior. Determining the most favorable setting will likely require some experimentation; if you have InterSystems IRIS deployed on a Linux/NUMA system, InterSystems recommends that you try an initial value of 250.